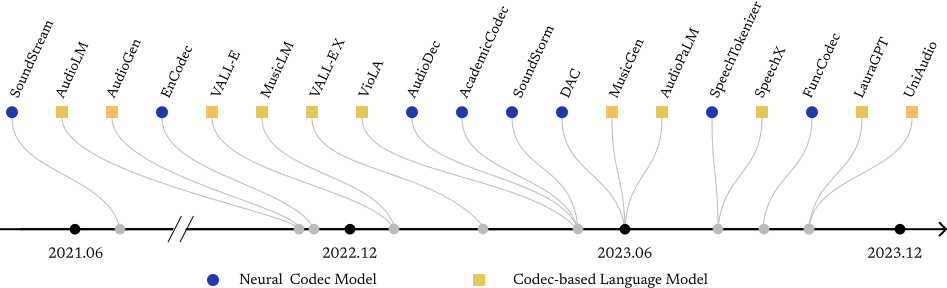
Introduction
Neural audio codecs are initially introduced to compress audio data into compact codes
to reduce transmission latency. Researchers recently discovered the potential of codecs
as suitable tokenizers for converting continuous audio into discrete codes, which can be
employed to develop audio language models (LMs). The neural audio codec's dual roles in
minimizing data transmission latency and serving as tokenizers underscore its critical
importance. The ideal neural audio codec models should preserve content,
paralinguistics, speakers,
and audio information. However, the
question of which codec achieves optimal audio information preservation remains
unanswered, as in different papers, models are evaluated on their selected experimental
settings. There's a lack of a challenge to enable a fair comparison of all current
existing codec models and stimulate the development of more advanced codecs. To fill
this blank, we propose the Codec-SUPERB challenge.
The goal of this challenge is to encourage innovative methods and a comprehensive
understanding of the capability of codec models. This challenge will conduct a
comprehensive analysis to provide insights into codec models from both application and
signal perspectives, diverging from previous codec papers that predominantly focus on
signal-level comparisons following the paper
Codec-SUPERB: An In-Depth
Analysis of Sound Codec Models (Wu et al., arXiv 2024). The diverse set of
signal-level metrics,
including Perceptual Evaluation of Speech Quality (PESQ), Short-Time Objective
Intelligibility (STOI), Mel distance, and Signal-to-Distortion Ratio (SDR) enable us to
conduct a thorough evaluation of
sound
quality across various dimensions, encompassing spectral fidelity, temporal
dynamics,
perceptual clarity, and intelligibility. The application angle evaluation will
comprehensively analyze each codec's ability to preserve crucial audio information,
encompassing content, speaker timbre, emotion, and general audio characteristics. We
hope this challenge can inspire innovative research in neural codec development.
With
this proposal, we aim to promote innovation in neural audio codec fields and
advancing
the research frontier.